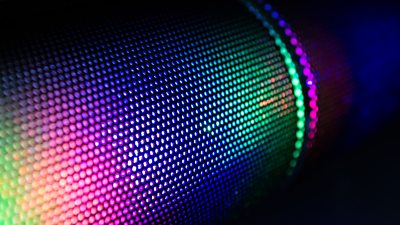
Recent advances in deep learning have enabled the automatization of many traditional production tasks that have the potential to transform the way 大象传媒 makes its programmes. Here at 大象传媒 Research & Development, we are researching how the quality of video could be enhanced by artificial intelligence and in particular how video can be automatically colourised using some of the most recent breakthroughs in machine learning. As a result of our research, we are proposing a new and original algorithm that is capable of performing this task even more efficiently, making images and videos look more colourful and realistic.
We recently developed a system to enhance the quality of user-generated video content using deep learning algorithms. Inspired by this, we have begun to investigate how to automatically enhance the colour of visual content using some of the most recent breakthroughs in machine learning. Adding colour information to images has become an area of signi铿乧ant interest for many, including within the broadcasting sector where this approach can be especially beneficial for the restoration of archive material.
An Open Source software is now available on this work via the . You can also , presented in - a Multimedia Signal Processing workshop from the .
Colourisation refers to the process of adding colours to greyscale or other monochrome images so that the coloured results are perceptually meaningful and visually appealing. The Canadian Wilson Markle introduced a novel computer-assisted technology for adding colour to black and white movies and TV programs in 1970. Although this improved the efficiency of traditional hand-crafted techniques, it still required a considerable amount of manual effort and artistic experience to achieve acceptable results. It has subsequently been shown that the task is complex and results could actually bear little resemblance to the colours in real life due to the large degrees of freedom possible in the task. For example, an algorithm could interpret rapid changes in a scene as an area of vegetation, assigning green colours to it, or smooth areas to the sky, inferring blue tones. In most cases the decisions on colouring are ambiguous - no rule directly determines a car to be red, blue or yellow without possessing additional knowledge of the scene as it was filmed in real life.
Greyscale content is present in a wide variety of circumstances: from faded 鈥渂lack and white鈥 archive material, to (video tracking or object recognition, for instance) where colour in the picture is discarded to simplify processing. However, while the brightness in the image helps us interpret shapes and structures in the image, the perception of colour is very important for modern video viewing. It is also essential for understanding the visual world, creating a greater distinction between objects and adding physical variations, such as shadows, reflections or reflectance variations on video frames. For this reason, adding colour information to images and improving the quality of colour has become a research area of significant interest for a wide variety of situations that traditionally have resorted to using luminance data alone. This includes medical imaging, surveillance systems or restoration of degraded historical images.
Since the 1970s however, the technology has improved considerably, resulting in some remarkable video restoration achievements. A successful example is film director Peter Jackson鈥檚 critically acclaimed World War I documentary, They Shall Not Grow Old in which modern restoration techniques were used to colourise original footages during the conflict, provided by 大象传媒 Archives and the .
Recently, advances within artificial intelligence (AI) have enabled the development of new colourisation algorithms based on deep learning. These Generative Adversarial Networks (GANs) are better at colouring natural images, leading to more realistic and plausible results and they have become the standard for many image-to-image translation tasks such as generating realistic street scenes from semantic segmentation maps, aerial photography from cartographic maps, and, in our case, image colourisation.
Our colourisation GAN is an adversarial algorithm which comprises two AI systems called 鈥済enerator and discriminator鈥. The generator tries to produce realistic (but fake) colours from a black and white image, while the discriminator acts as a judge and tries to identify whether the results are fake or not. A competition takes place as the generator then tries to get better at producing realistically coloured images, and the discriminator gets better at detecting fake images. This video shows the generator competing with the discriminator to colourise greyscale pictures.
After achieving promising results with still images, we are now aiming to adapt our system to more realistic broadcasting uses, addressing any inconsistencies when applying our research on video footage. We also want to move more of our research into 鈥榮tyle transfer鈥 鈥 improving results by transferring colour information from other similar material. For instance, if we have a black and white image of a car in a forest, we could transfer over colour information from a frame in a similar coloured image. In this example, a red car in a forest on a cloudy day would be a point of reference for our algorithm and would copy over the colour of the vehicle, plus grey colours for the sky, dark colours for the lighting and so on. This kind of style transfer reduces the ambiguity of the colours chosen by the algorithm.
- This work was carried out within the in collaboration with the and the .