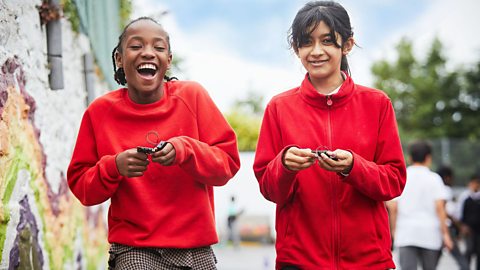
Back in 2015, the 大象传媒 micro:bit was created to help pupils understand the world of coding in a tangible, fun way. Today, the micro:bit is yet again helping pupils get to grips with a tricky computing concept - machine learning - and giving them a practical way to explore this in their very own playground as part of the 大象传媒 micro:bit 鈥 the next gen campaign. Here, the Micro:bit Educational Foundation鈥檚 Bl谩thnaid Walsh-Heron explains how the project came together鈥
Exploring how machine learning could work on the micro:bit
In late 2022 the Micro:bit Educational Foundation started to explore how the features of the micro:bit could be used to bring the concept of machine learning (ML) to life for primary pupils. ML is an application of AI (artificial intelligence) technology where computer software is designed to perform a task quickly and reliably, having been trained by examples of data provided by humans. Put simply, it involves collecting data in order to train a computer to do something.
Teaching children about the increasing importance of data in today鈥檚 world, and helping pupils understand how this links to AI would sound daunting to many primary teachers. However, the team at the Micro:bit Educational Foundation were confident that putting an ML model on the micro:bit, and allowing pupils to use, interact and rate it in their school environment, would help both teachers and pupils make sense of this concept in a practical and visual way.
Two of the micro:bit鈥檚 data logging features stood out as ways of engaging learners around machine learning: sound data collected using the micro:bit鈥檚 microphone and movement data collected using the accelerometer (movement sensor). Both could be used to train a machine learning model that detected either voice or movement with the micro:bit.
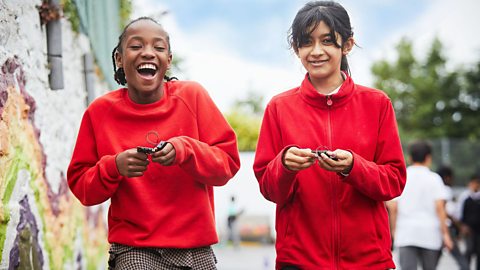
Investigating movement data to train a model
The team at the Micro:bit Educational Foundation chose to explore using movement data from the micro:bit鈥檚 accelerometer as it aligned with work that had already begun on the 大象传媒 micro:bit playground survey. The survey activities use the micro:bit as a tool to gather data from school playgrounds. Developing a micro:bit activity tracker trained using ML, similar to a Fitbit or smart watch, meant learning about ML could be included in the playground survey suite of activities.
In addition, the Foundation team saw the effectiveness of a piece of research and development carried out by Aarhus University in Denmark. They had used motion samples collected live in the classroom to train a machine learning model in a way that reduced complexity and made it interactive and fun. This showed that logging and visualising movement data was an ideal gateway to teaching ML to primary age children.
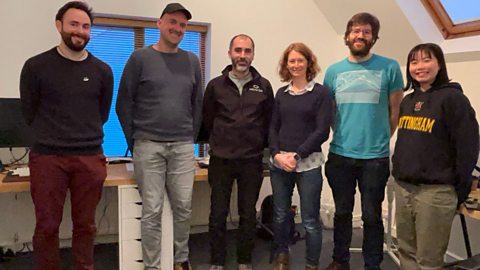
First steps and a little help from the experts
To create a good model, you need a lot of training data. Starting with a publicly available dataset, the team began to work on the model alongside Neil Tan, a machine learning developer at . The data set, called 鈥榮elfBACK鈥, is a Human Activity Recognition Dataset, and it included samples of wrist-worn accelerometer data, which was similar to data from micro:bits worn on wrists.
A lot of help from pupils across the UK
In order to improve how the model detected activity in the playground, more specific data samples were needed. The more training data available, the more accurate the model would be. It was also important to have data that matched the specific activities that would be detected. Primary pupils from Northern Ireland, Scotland, Wales and England helped collect samples of data that were used to train, test and improve the model. This data included movements that often happen in playgrounds such as walking, running and having a chat with friends.
Improving accuracy
Creating an ML model that detects children鈥檚 activity and that runs on a micro:bit had never been done before. The team faced a number of challenges to get the activity tracker working as accurately as possible.Children move in lots of different ways, even when asked to 鈥榬un鈥 or 鈥榳alk鈥. The team learnt in later data collections to ask pupils to move in very specific ways to ensure every possible variation existed within the training data. For example, run in a straight line or run clockwise in a circle.
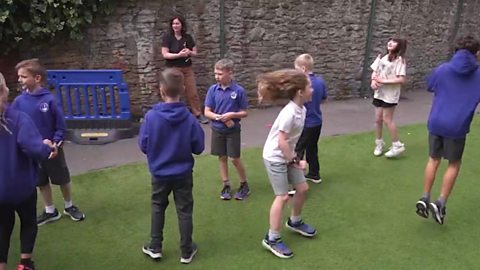
Initially data was collected for games such as 鈥榮tuck in the mud鈥 and 鈥榯ag鈥. It became clear though that the model was confused by data like this. These games consist of other activities in sequence, for example, running, then being still, then walking, then running again. Each sequence needed to be chopped into smaller segments for training, and this made it difficult for the model to identify 鈥榮tuck in the mud鈥 from the other activities it contained. It was decided that when children viewed their activity data, they would be able to identify when they were playing a game by the sequence of activities and so 鈥榞ames鈥 were removed from the training data.
The activity tracker is created
The development work resulted in a self-contained program that turns a micro:bit into an activity tracker. Pupils transfer the program onto the micro:bit, then wear it on their wrist in the playground. The accelerometer within the micro:bit detects the movement currently happening, and the ML model runs simultaneously estimating the actual activity taking place. This means pupils can wear it and then analyse their movement data on graphs to see how much time they spent doing different activities such as running, walking, chatting etc.
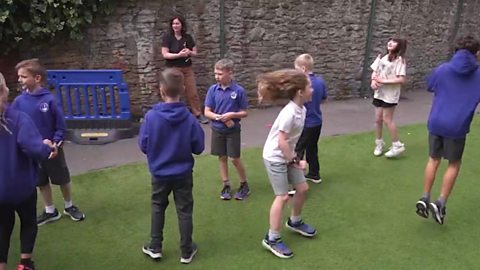
Teachers and pupils test it out
Testing began with small groups of learners wearing the micro:bit (programmed with the model) on their wrist while they played outside. The wrist-worn micro:bits sent the activity estimates to another micro:bit by radio. This meant the team could make real-time comparisons between how they observed pupils moving and what the model was estimating pupils were doing.
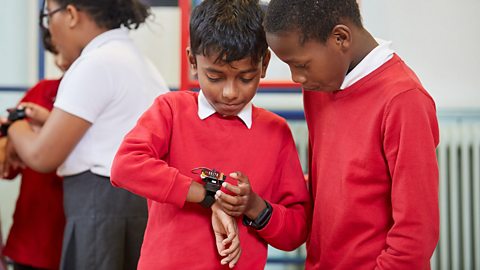
Whole class testing then took place where the pupils wore the micro:bit for longer periods of time and viewed graphs of their movement data to see if the activity tracker was accurately detecting their activity.
Lastly, the team also created and tested an alternative program that logs and displays only the accelerometer data. This was to ensure that all pupils, including those that did not usually run, jump or walk in their playground, could take part in the activity and analyse their movement graphs.
Empowering children for the future
The activity tracker model has now launched as part of the 大象传媒 micro:bit playground survey and pupils all over the UK will be able to explore machine learning in the classroom.
Jonny Austin, Chief Technology and Product Officer at the Micro:bit Educational Foundation, said:
鈥淎I is transforming the way we live, learn and work. Helping children to understand what computers can, can鈥檛 and could do will empower them to shape the way technology is used in our society. By building this model that allows students to experience and understand machine learning, we support them in designing future AI-enabled solutions for the things they think are important, making it more inclusive, equitable and for the benefit of wider society.鈥
To find out more about using the activity tracker within the 大象传媒 micro:bit playground survey visit the activity page.
More on the playground survey
Get pupils to use the special micro:bit Activity Tracker program to track their physical activity in their school playground.
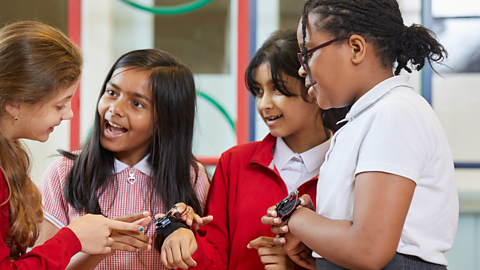
Explore how computers learn from data using the new micro:bit machine learning tool. Have fun training your own machine learning model!
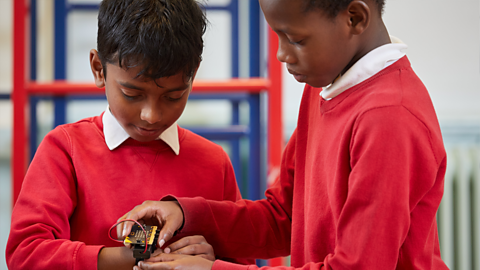
Find out everything you need to know about the playground survey and download all of the learning resources available.
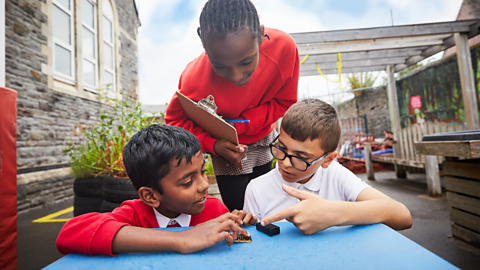