Lighting is a powerful tool in TV and film production. in a scene can create a wide range of moods, which can enhance and alter how the story is told.
Nikolina Kubiak, a PhD student from the , is collaborating with ´óÏó´«Ã½ Research & Development to explore how video could be captured under any available lighting (even if this is not ideal) and then automatically altered in post-production to the style the producer wants.
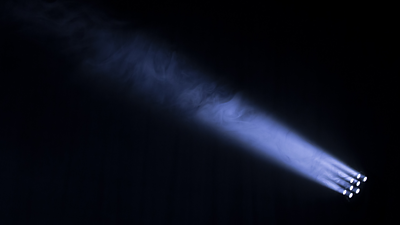
News broadcasts or studio dramas have the lighting they need permanently installed, operated by crews who can automate some of the lighting effects. Outside of the studio, however, it can be a different story. Some large events, such as music festivals, have dedicated crews and attract large audiences, making professional lighting possible and justifying the significant effort and costs of covering the event.
For remote venues or smaller-scale comedy, music, or other cultural and educational events, there may not be the facilities, kit, crew, or transportation to light an event professionally. However, these events could still interest many viewers, are just as valuable and deserve to be seen.
To try and open up these new opportunities, we're investigating whether it's possible in post-production to automate the re-lighting of footage for events that don't have a dedicated lighting crew.
Our research uses deep-learning techniques to create a machine learning model capable of generating new versions of a scene as if we captured it under a specified fixed lighting style. We have created a network called SILT (Self-supervised Implicit Lighting Transfer) which 're-styles' input data captured under unspecified lighting conditions to a lighting style we have specified. .
The example above shows . On the left is a scene in arbitrary lighting conditions, and on the right is a reference image of the scene under the lighting style we are trying to match. The middle picture is the same as the one on the left but re-styled to match the fixed target lighting style images on the right. You can see the right side of the dune is lit up, mimicking our target illumination conditions. However, this re-stylisation is imperfect as we can see differences between the output and the reference data. This happens because the lighting effects in the input images have not been entirely removed, so they still affect the scene's appearance. We hope to correct this issue as we optimise the model further.
Our proposed solution uses a Generative Adversarial Network (GAN) to re-style the scenes. GANs are made of two key model parts – the generator and the discriminator. Imagine the generator as a forger trying to make realistic-looking images. The discriminator works like a detective – it sees the fake images created by the generator and real images of scenes which look similar - and learns to distinguish between them. As the discriminator gets better at recognising the fake samples, the generator has to get better at forging to produce images that closely resemble real-life pictures. Through this adversarial game, the performance of both components improves, and the system generates more convincing results. The SILT GAN outputs images re-styled to mimic the desired lighting conditions, and the discriminator compares them with real images depicting the same lighting style.
- ´óÏó´«Ã½ R&D - AI & Auto colourisation - Black & white to colour with machine learning
- ´óÏó´«Ã½ R&D - Visual data analytics
Machine learning models like these learn by finding patterns and correlations in large amounts of training data. Most existing systems are supervised, i.e. their learning is controlled by comparing the model output with a paired reference. The error calculated between these two samples is then used to update the internal parameters of the system in the hope of improving the 'correctness' of the future outputs.
Unfortunately, it is not always easy to collect this type of data. Photographing one person in a fixed environment under a few different lighting conditions is relatively easy. However, capturing a live event under many different illumination settings is impracticable. The capture area is much larger, difficult to constrain, and there are usually many moving elements in the scene, such as people.
This is where self-supervised learning is valuable. No longer requiring aligned reference data, self-supervised models rely on the information contained in other examples from the training dataset, none of which have to be the reference. Consequently, we used this versatile approach while designing SILT. When training the model, SILT never has to see examples of our scene lit the way we intend audiences to see it before it carries out any re-styling. Instead, SILT works out the characteristics of our intended lighting style from a collection of images showing arbitrary scenes lit similarly.
This flexibility means our system can infer lighting characteristics from the rich collection of content in the ´óÏó´«Ã½'s archives. For example, the ´óÏó´«Ã½ has recorded many shows using the , with little-to-no shadow on the participants' faces. In the future, archive material with this type of illumination could be used as a style reference to train the model to re-style other similar content.
Our system is still a work in progress. SILT has no explicit depth perception and can't differentiate between objects within the scene. With added scene understanding, we hope the model could learn to distinguish between constant or varying scene components and, as a result, manipulate the lighting more accurately. ´óÏó´«Ã½ has focused on still images so far, but we intend to expand SILT to work seamlessly with video and hope to integrate the algorithms developed during this studentship with Ed, ´óÏó´«Ã½ R&D's automated video editing tool.
- -
- ´óÏó´«Ã½ R&D - AI in media production
- ´óÏó´«Ã½ R&D - AI Opportunities: Transforming Coverage of Live Events
- ´óÏó´«Ã½ R&D - Faster video compression using machine learning
- ´óÏó´«Ã½ R&D - AI & Auto colourisation - Black & white to colour with machine learning
- ´óÏó´«Ã½ R&D - Visual data analytics
- ´óÏó´«Ã½ R&D - DVC-P: Deep video compression with perceptual optimizations
- ´óÏó´«Ã½ R&D - Reimagining image compression in the era of machine learning
- ´óÏó´«Ã½ R&D - A new dataset to improve TV production using artificial intelligence and machine learning